- AI Blog Project control Project Management
- 20 Feb 2025
AI for EPC Project Management: Demystifying Common Myths
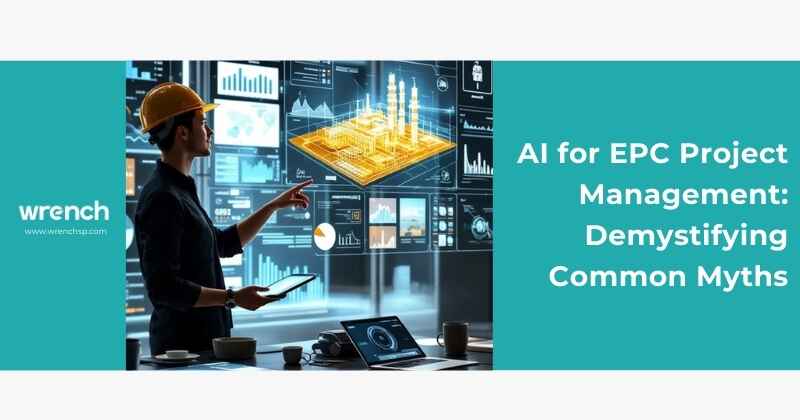
AI is being aggressively rolled out in a wide range of industries from Healthcare to Banking to Retail, but the EPC sector seems reluctant to join in. That reluctance, in many cases, stems from widely held misconceptions and myths about AI in the context of EPC project management. In this series we’ll be leveraging our experience of consulting with EPC organizations around the world, and with project control teams, to dispel some of the most popular myths.
We shall focus our discussions in this series on the top three myths as below;
Myth 1 – You need highly structured and large volumes of data to implement AI
Myth 2 – You won’t see immediate benefits from AI; it takes years of training for AI to be effective
Myth 3 – Your confidential intellectual property will end up being shared in the public domain
In this post we’ll address the first myth.
Myth: AI needs structured and large datasets to be effective
We found this to be a very common idea, which on investigation seemed to have originated from the real-life historical and functional challenges that Project Control professionals face. Because, of course, the data is fragmented and not structured; it originates from multiple sources – cost control systems, scheduling tools, procurement logs, and contractor reports – and all those datasets tend to be inconsistent, fragmented, or misaligned with each other. This was aggravated by the fact EPC organizations have historically relied very heavily on manual processes for cleaning, consolidating, and analyzing data for their reports and forecasts. It’s not surprising that a belief arose that such data would be unsuitable to ‘advanced’ technology like AI, although this couldn’t be further from the truth as we’ll see shortly.
Another, related, assumption, was that implementing AI would be a complicated undertaking because the company would have to align their legacy project data formats across tools like Primavera P6, MS Project, and Excel; not to mention data from external vendors and contractors. Companies found it hard to envisage AI functioning efficiently without first perfectly aligning all these tools and their data.
We also found a surprising lack of awareness about modern AI Capabilities, likely stemming from the fact that traditional processes used by Project Control teams such as, the earned value management, cost tracking, or schedule analysis, etc., tend to rely very heavily on structured datasets. So, it is only reasonable for these teams to assume that AI requires the same level of structure and detail. This misconception unfortunately has only gained strength with time.
Now let’s pivot from the myth to the fact.
Fact: AI thrives on Unstructured and Incomplete Data.
Unlike traditional tools, today’s AI is designed to process and extract insights from unstructured or semi-structured data, such as progress reports, meeting minutes, and even scanned PDFs. For instance, Natural Language Processing (NLP)-based AI can analyze contractor reports or change orders to identify potential schedule impacts or cost implications, even if the data isn’t fully standardized. AI can even extract key metrics from diverse sources—like combining procurement schedules with field updates—without needing them to be in a uniform format.
Pre-trained AI models can start delivering value with smaller datasets, by leveraging industry knowledge already embedded in the system. That is to say, an AI scheduling tool does not require access to every project schedule ever created by the company; it can work with a limited set of historical schedules to identify patterns like recurring delays in critical path activities or resource shortages during peak construction phases.
AI Tools can also work Incrementally i.e. they can generate meaningful insights with just the data currently available and then keep improving the accuracy of those insights as more data gets added over time. So, you could have an AI-powered forecasting tool that starts with a subset of historical cost data to predict overruns in procurement but continue to refine its accuracy as more project data is fed into the system during execution.
In the same way AI can provide early risk forecasts based on partial progress data and then refine its predictions as additional updates get integrated.
AI also excels at data integration across formats, in fact today’s AI systems are designed to integrate data from various sources, including scheduling software, cost control systems, and field reports, using advanced algorithms to identify correlations even when datasets are incomplete or differently formatted. So, your AI would be able to combine Primavera P6 schedules with Excel procurement logs and IoT sensor data from the field to flag schedule risks, such as delays in material delivery affecting critical activities.
In other words, your AI would give you real-time Insights from your current data and live data streams; it doesn’t require years of historical data to begin providing actionable insights; it can work with daily progress updates or cost transactions to identify trends and optimize decision-making in real time. For example, your AI-powered dashboards would highlight discrepancies between planned and actual progress based on weekly site updates and without waiting for a full dataset.
Real-World Applications of AI in Project Controls
Now that we know AI doesn’t need structured or complete datasets to provide value, let’s explore some of its real-world applications in Project Controls.
Progress Tracking and Validation: AI tools can analyze daily site updates, progress photos, or drone footage to validate reported progress against actual field conditions.
Schedule Risk Analysis: AI can identify high-risk activities in a project schedule by analyzing limited historical performance data or live updates from ongoing work.
Cost Anomaly Detection: AI can flag unusual cost patterns in real time, such as sudden spikes in procurement costs or labor hours, without relying on a comprehensive historical cost database.
Forecasting: AI tools can provide accurate earned value forecasts using a combination of live and historical data, helping teams identify potential budget and schedule overruns early.
Conclusion: A Tip for Project Controls Teams hesitant about AI.
We suggest companies worried about the quality of their data being a roadblock to AI adoption start small, by applying AI to targeted use cases where unstructured or incomplete data is the norm. For example: they could use AI to validate progress based on unstructured reports or images, they could use it to combine and analyze cost data from fragmented sources, or they could use it to flag schedule risks by integrating data from scheduling software and contractor reports. By seeing the effect of AI on such specific and high-impact applications, the myth about it needing perfectly structured or vast amounts of data would be soon dispelled.
In the next article we tackle Myth 2 – No Immediate benefits from AI: It takes years of training for it to be effective.
With over 25 years of experience in the energy and maritime industries, Chimbu has been at the forefront of driving transformational changes and enhancing business processes. Prior to joining Wrench, he spent 10+ years at Petrofac, including serving as Head of Project Control & Systems.
Related Posts
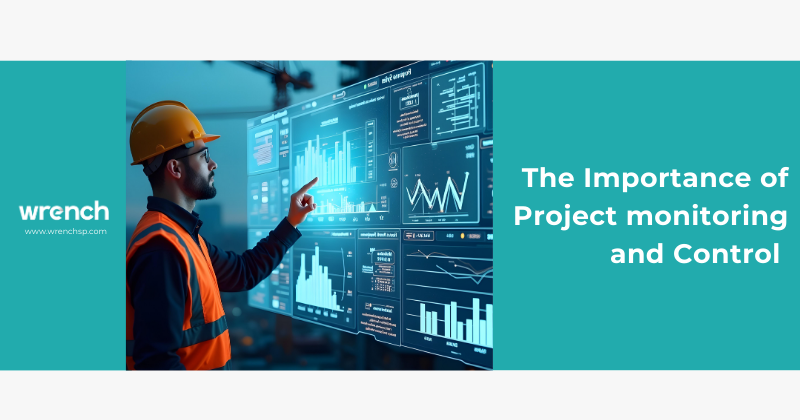
The Importance of Project Monitoring and Control
The ability to monitor and track progress in EPC projects is essential to its successful delivery. That means being able to implement reliable project monitoring systems that will let you measure and document how work…
- 21 Feb 2025
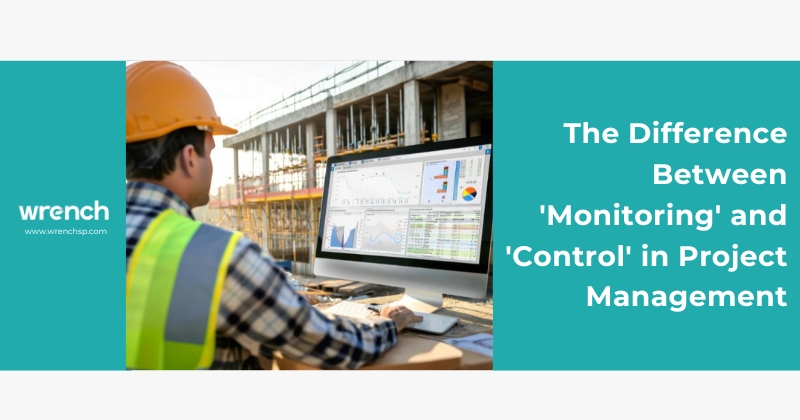
The Difference Between Monitoring and Control in Project Management
When EPC projects fail, there are a number of factors that contributed to that failure, and when companies attempt a post mortem, various inefficiencies in project management tend to crop up as the cause. However,…
- 06 Feb 2025
Archives
- February 2025
- January 2025
- November 2024
- October 2024
- September 2024
- August 2024
- July 2024
- June 2024
- May 2024
- April 2024
- March 2024
- January 2024
- December 2023
- November 2023
- September 2023
- August 2023
- July 2023
- June 2023
- May 2023
- April 2023
- March 2023
- February 2023
- January 2023
- December 2022
- November 2022
- September 2022
- June 2022
- May 2022
- April 2022
- March 2022
- January 2022
- November 2021
- October 2021
- July 2021
- June 2021
- May 2021
- March 2021
- February 2021
- January 2021
- December 2020
- November 2020
- September 2020
- August 2020
- June 2020
- April 2020
- March 2020
- February 2020
- January 2020
- November 2019
- October 2019
- September 2019
- August 2019
- April 2019
- March 2019
- December 2018
- October 2018
- September 2018
- August 2018
- July 2018
- June 2018
- May 2018
- April 2018
- January 2018
- November 2017
- October 2017
- September 2017
- May 2017
- April 2017
- March 2017
- February 2017
- January 2017